Record Detail
Advanced Search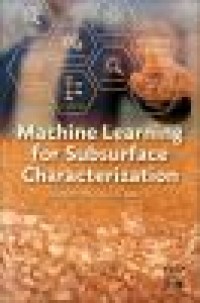
Text
Machine learning for subsurface characterization
Machine Learning for Subsurface Characterization develops and applies neural networks, random forests, deep learning, unsupervised learning, Bayesian frameworks, and clustering methods for subsurface characterization. Machine learning (ML) focusses on developing computational methods/algorithms that learn to recognize patterns and quantify functional relationships by processing large data sets, also referred to as the "big data." Deep learning (DL) is a subset of machine learning that processes "big data" to construct numerous layers of abstraction to accomplish the learning task. DL methods do not require the manual step of extracting/engineering features; however, it requires us to provide large amounts of data along with high-performance computing to obtain reliable results in a timely manner. This reference helps the engineers, geophysicists, and geoscientists get familiar with data science and analytics terminology relevant to subsurface characterization and demonstrates the use of data-driven methods for outlier detection, geomechanical/electromagnetic characterization, image analysis, fluid saturation estimation, and pore-scale characterization in the subsurface.
Availability
9620/PUP/2020 | 808.6 MIS m c.1 | Perpustakaan Universitas Pertamina | Available |
9622/PUP/2020 | 808.6 MIS m c.2 | Perpustakaan Universitas Pertamina | Available |
Detail Information
Series Title |
-
|
---|---|
Call Number |
808.6 MIS m
|
Publisher | Gulf Professional Publishing : England., 2020 |
Collation |
xxvii, 442 pages, illust 23 cm
|
Language |
English
|
ISBN/ISSN |
9780128177365
|
Classification |
808.6
|
Content Type |
-
|
Media Type |
-
|
---|---|
Carrier Type |
-
|
Edition |
-
|
Subject(s) | |
Specific Detail Info |
-
|
Statement of Responsibility |
-
|
Department(s) |
-
|
Other version/related
No other version available